Real-Time Fraud Detection for Contact Centers
Fraud threatens fintech and banks, with risks like social engineering and payment fraud rising due to digital banking. Rulebase Radar provides real-time fraud detection for contact centers, using AI risk scoring and behavioral analysis to enhance security across voice, email, and messaging.
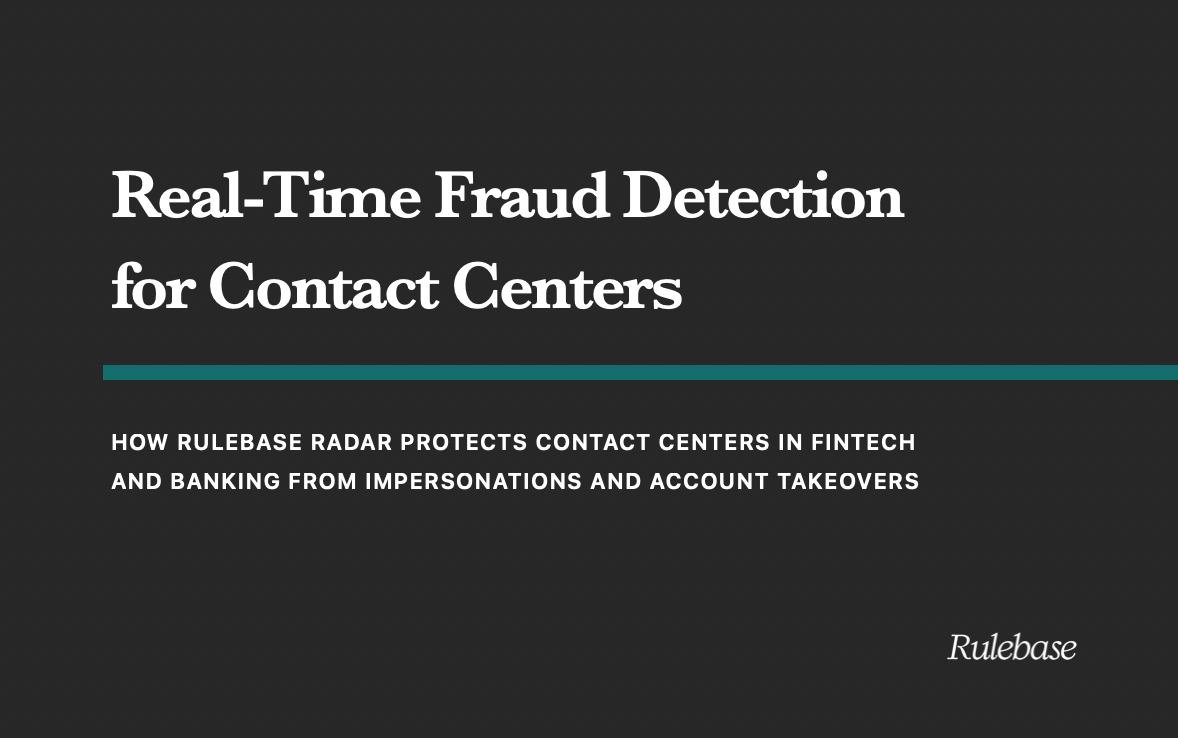
Rulebase Radar: Fraud Prevention for Fintech and Banking
The digital financial ecosystem is highly vulnerable to fraud, requiring robust detection systems. Fintech platforms handling high-value transactions must ensure secure, compliant, and fraud-free operations. Traditional fraud detection methods often fail due to:
- Increasingly sophisticated fraud tactics
- Inadequate real-time intervention
- High false positive/rates impacting customer experience
Rulebase Radar addresses these challenges with a multi-layered risk assessment approach, enabling fintechs to:
- Detect fraud attempts in real time
- Minimize financial losses due to fraud
- Enhance customer trust with secure transactions
Unlike traditional fraud detection solutions that rely heavily on static rule-based systems and reactive analysis, Rulebase Radar employs an AI-driven, real-time risk assessment approach. Many existing systems fail to detect evolving fraud patterns due to their dependence on historical data and manual intervention. Rulebase Radar stands apart by leveraging real-time call analysis, behavioral biometrics, and machine learning to detect fraud attempts before they escalate. Its seamless integration with CCaaS platforms allows instant fraud detection without disrupting customer service workflows, making it a more efficient and scalable solution than legacy fraud detection methods.
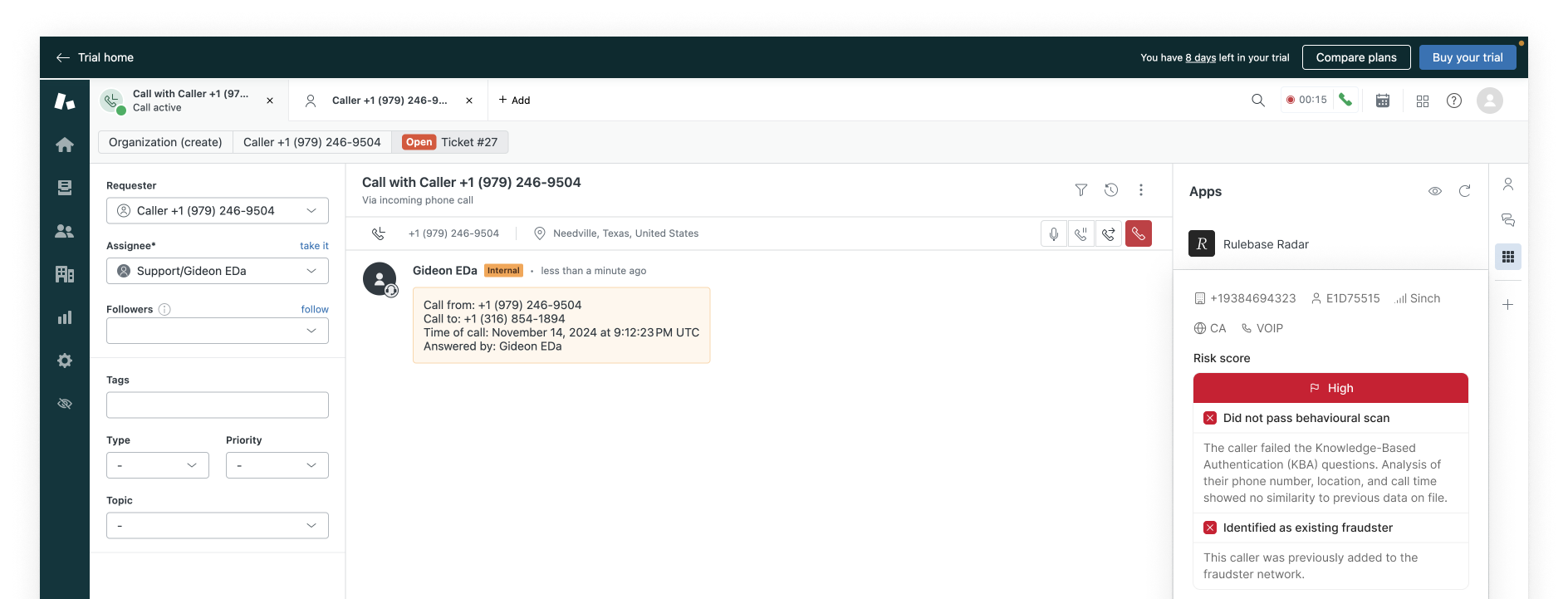
Risk Scoring Model
Rulebase Radar employs a comprehensive risk-scoring model to analyze incoming and ongoing calls. The system evaluates risk indicators across two main categories:
Phone Number and Network Analysis
Rulebase Radar assesses caller identity through both phone number reputation and network risk scoring.
Phone Number Analysis
The system searches incoming phone numbers against third-party fraud databases, identifying numbers previously flagged for fraudulent activity. Additionally, Rulebase Radar maintains a proprietary database of fraudulent calls, continuously updated to reflect emerging threats. This dual-layered approach enhances fraud detection accuracy, allowing financial institutions to proactively block high-risk callers before they initiate fraudulent transactions.
Network Risk Scoring
Rulebase Radar also evaluates the network used to place the call, flagging high-risk caller networks commonly associated with fraud. This includes VoIP-based systems, which are frequently exploited by fraudsters due to their anonymity and ease of manipulation. Calls originating from suspicious or unverified VoIP networks are assigned a higher risk score, enabling proactive fraud prevention strategies. By combining phone number reputation with network risk analysis, Rulebase Radar significantly improves fraud detection and reduces financial losses for fintech companies and banks.
Behavioral Pattern Analysis
Rulebase Radar monitors deviations in customer call behavior to flag anomalies that may indicate fraudulent intent. Key areas of analysis include:
Social Engineering Detection
Fraudsters often use social engineering tactics to manipulate customer service representatives. Rulebase Radar detects suspicious conversational patterns, such as excessive persuasion, emotional manipulation, and fabricated urgency, signaling potential fraud attempts.
NLP-Based Urgency and Aggression Detection
Using natural language processing (NLP), Rulebase Radar analyzes speech patterns for signs of urgency or aggression. Fraudsters frequently pressure agents into bypassing security measures by creating artificial emergencies or acting aggressively to rush authentication steps. The system flags such behaviors for additional scrutiny.
Resistance to Knowledge-Based Authentication Checks
Legitimate customers typically comply with knowledge-based authentication (KBA) procedures, while fraudsters attempt to evade them. Rulebase Radar tracks patterns of resistance, hesitation, or repeated incorrect answers during KBA verification, identifying high-risk interactions.
Similarity to Known Fraud Scripts
Fraud attempts often follow repeatable scripts. Rulebase Radar compares conversation structures against a database of known fraudulent scripts, identifying similarities in phrasing, tone, and tactics. This allows for the early detection of fraudulent intent before financial damage occurs.
Risk Calculation
Rulebase Radar assigns each call a real-time risk score by dynamically weighing multiple fraud indicators. The AI-driven risk model continuously adapts to emerging fraud tactics, ensuring high accuracy while reducing false positives. Risk levels are assigned based on thresholds.
The AI-driven risk model continuously adapts to emerging fraud tactics, ensuring high accuracy while reducing false positives. Risk levels are categorized into Low, Medium, and High, enabling fintech teams to act on suspicious interactions immediately. Thresholds and weightings are automatically recalibrated based on real-time fraud detection performance and historical accuracy.
System Integration
Ease of Integration
Rulebase Radar is designed for seamless deployment within existing fintech infrastructure. Its API-first architecture allows quick integration with customer service platforms, requiring minimal development effort. Pre-built connectors for leading CCaaS platforms further streamline implementation, enabling organizations to deploy fraud detection capabilities with minimal downtime.
Implementation Challenges
While integration is straightforward, fintechs may encounter challenges such as aligning risk-scoring models with existing fraud prevention strategies and ensuring compliance with industry regulations. To address these issues, Rulebase Radar provides detailed documentation, sandbox testing environments, and dedicated support to optimize configuration and performance.
Secure and Compliant Data Handling
Rulebase Radar processes only the essential data required for fraud detection, ensuring a balance between security and privacy. The system adheres to industry best practices, including:
- Minimal Data Collection: Only real-time call metadata and behavioral signals are used.
- Encryption & Access Controls: Data is secured through encryption and strict access policies.
- Compliance Alignment: Supports fintech compliance standards such as GDPR.
Conclusion
By implementing Rulebase Radar, fintech companies and banks can significantly reduce fraudulent transactions, improve compliance, and enhance the security of financial operations. Key benefits include:
- Reduced Fraud Losses: Real-time fraud detection prevents unauthorized transactions.
- Improved Customer Trust: Secure communication builds confidence among clients.
- Operational Efficiency: Automated risk assessment minimizes manual fraud investigations.
For fintech leaders, fraud prevention is not just a security measure—it’s a business imperative. Rulebase Radar provides an advanced, AI-driven solution that ensures financial integrity, customer protection, and operational efficiency. By leveraging cutting-edge fraud detection, fintech companies and banks can continue to lead the financial automation space with confidence and trust.
We invite fintech organizations to explore how Rulebase Radar can enhance fraud detection capabilities. Contact us today at founders@rulebase.co for a demo or consultation and take the next step toward a more secure financial ecosystem.